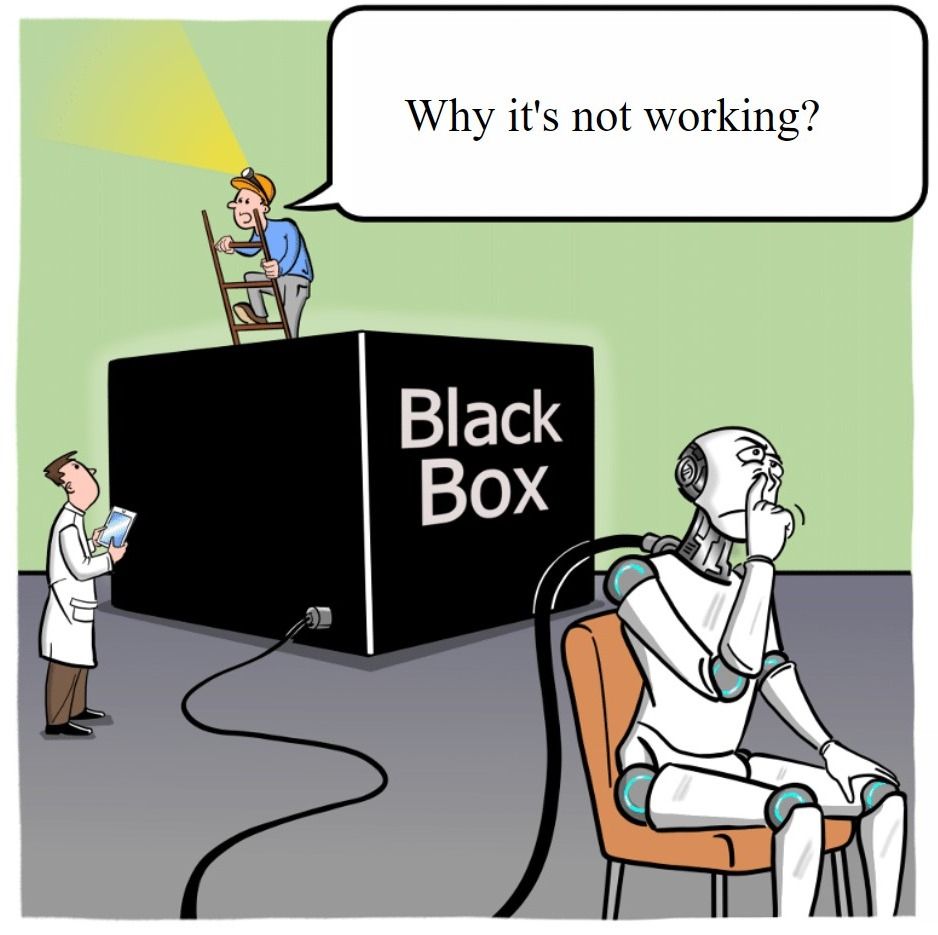
Supervisors
Authors
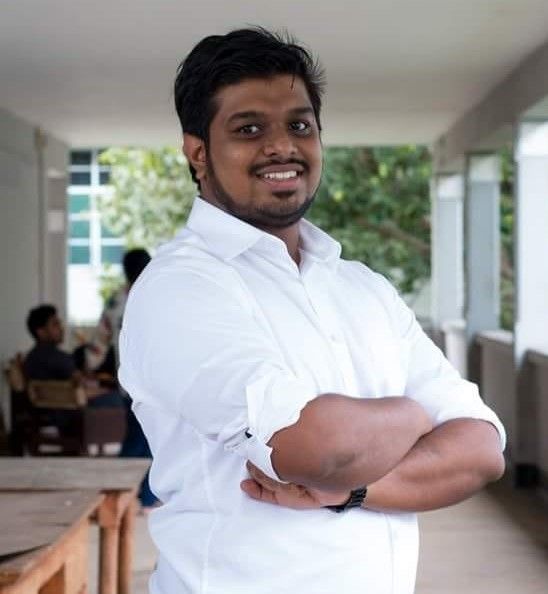
Imesh Ekanayake
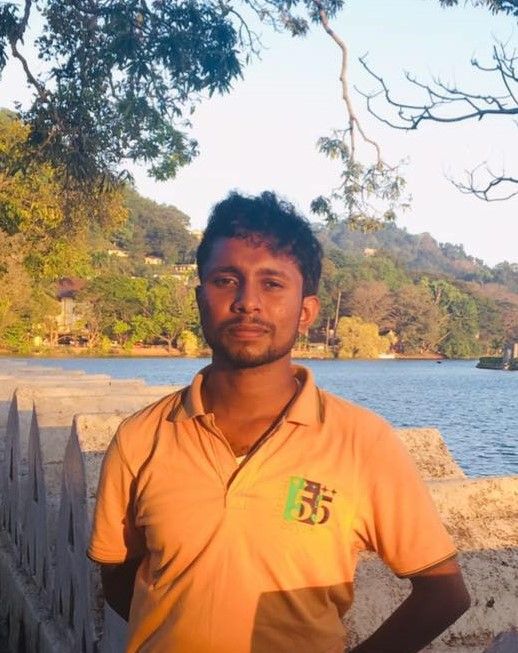
Machine Learning (ML) has contributed to many advances in science and technology. Recently a trend of applications in high-stake decision-making has been initiated. The advancement of ML made the decision-making process unclear with complex black-box models, especially the state-of-the-art models which have maximized the performance are more complex, inexplicable, and hard to explain. On the contrary, high-stakes settings as healthcare, finance, and criminal justice, have strict ethical concerns that made a mandatory requirement to explain each decision or the model as a whole. Besides, the acts and regulations like General Data Protection Regulation (GDPR) make it obligatory to explain the decisions made by computer systems and created a social right for explanations. One of the most pressing problems the field is explainability and interpretability of artificial intelligent systems. Moreover, it is necessary to ensure the fairness and transparency of a decision to obtain the stakeholders' trust. The theoretical knowledge of explainable machine learning is not well-tested on real-world problems with direct social impact. In this paper, we have identified a quandary that reflects the characteristics of a high-stakes machine learning problem in the public sector. A solution of an early warning system to predict the projects that could be unfunded in an educational crowdfunding platform in a resource-constrained environment has been presented.