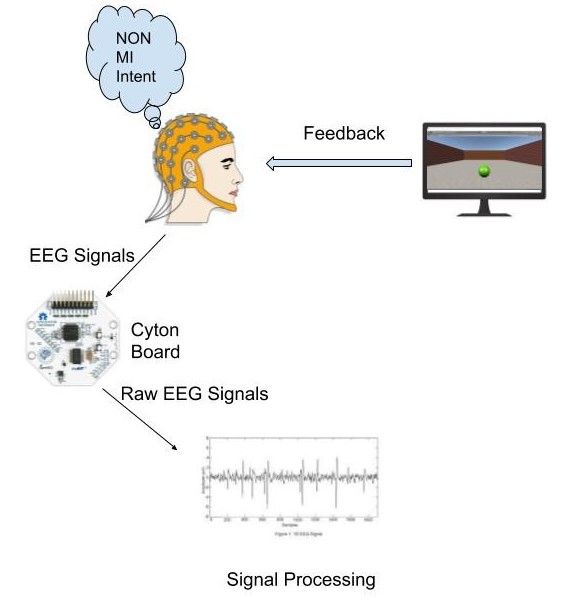
Supervisors
Authors
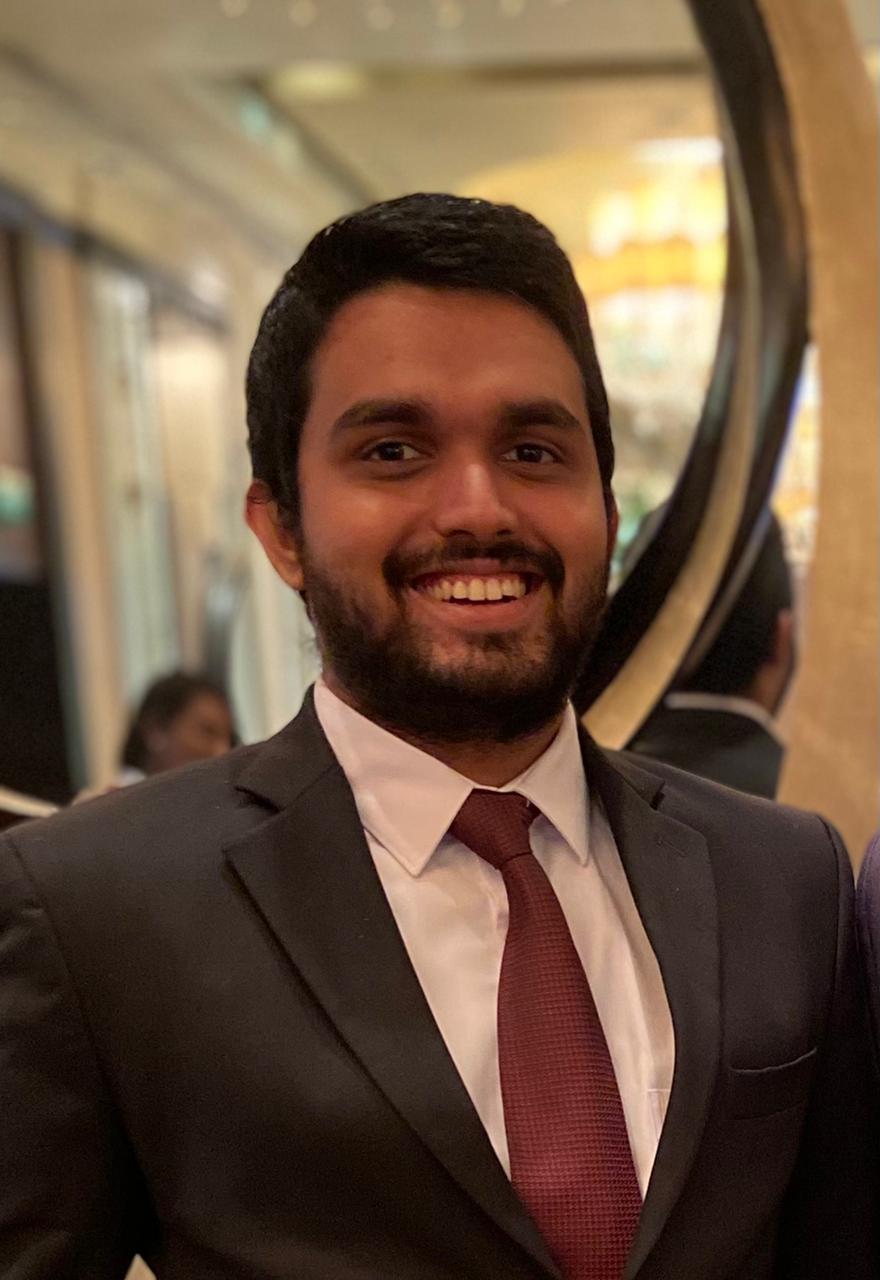
Prageeth Dassanayake
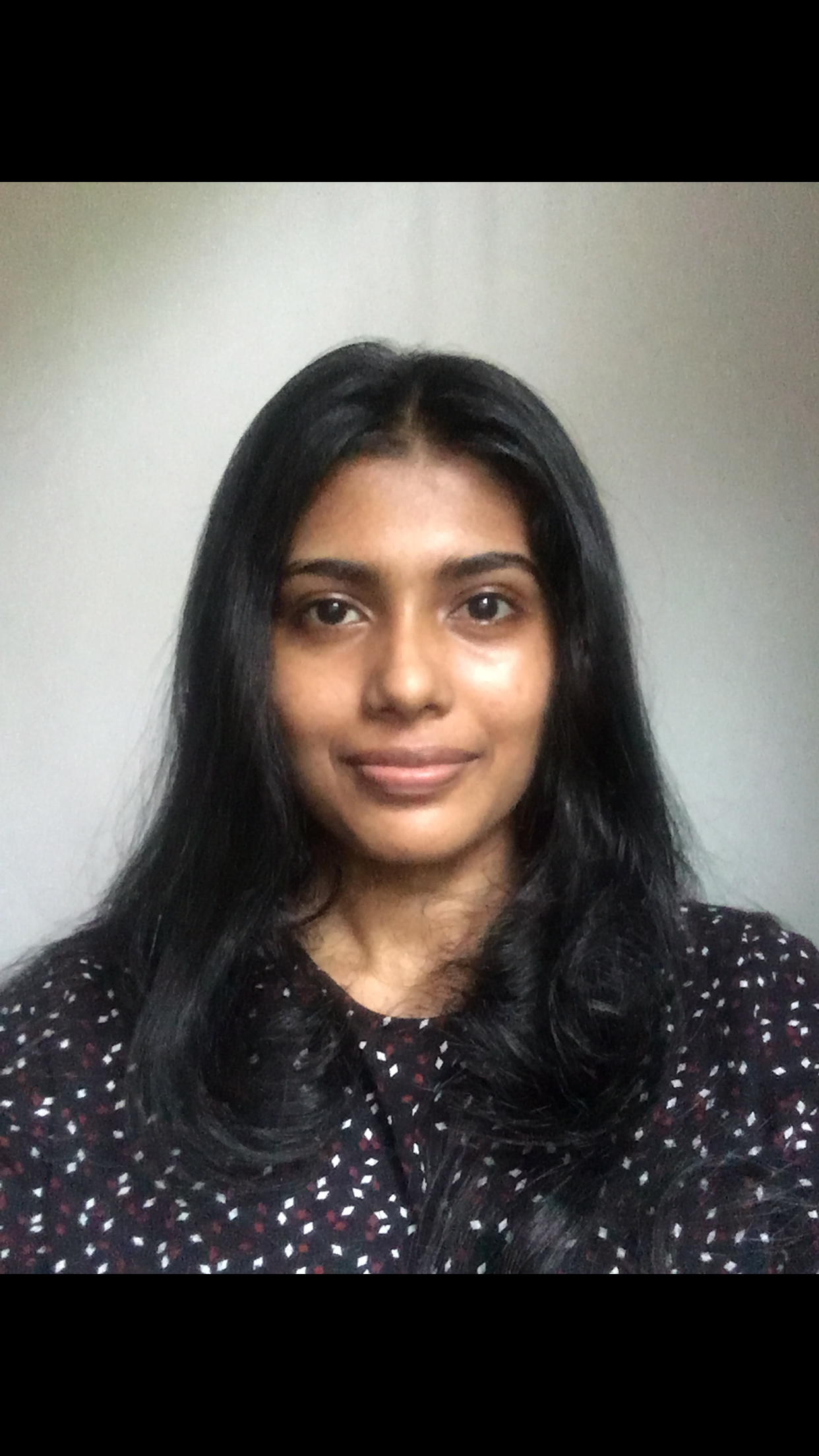
Sewwandie Nanayakkara
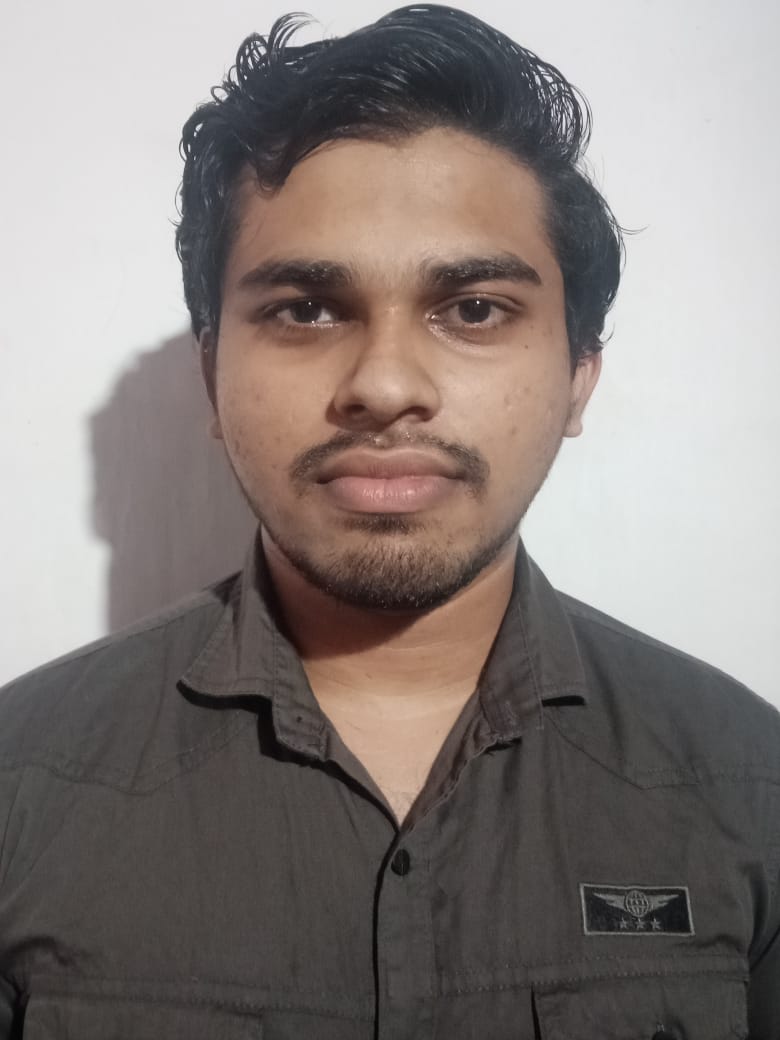
Non-invasive electroencephalogram (EEG) based Brain-Computer Interface (BCI) systems have been an interesting research area for many fields. However, most of the research done on this subject is synchronous, therefore the state of mind of the user is not similar to its natural behaviour. Considering to provide possible experience in practical applications, self-paced BCI systems started gaining popularity in recent years. However, there are certain challenges yet to be addressed when following this method. Out of the research done on self-paced BCI systems, most of them are focused on motor-imagery control whereas research on non-motor imagery mental tasks is limited. In this research, we analyse the possibility of using the techniques used in the motor-imagery method for non-motor imagery mental tasks to be fed into virtual object controlling applications. Research was done with 5 different classification models with the use of features from Fast Fourier Transform (FFT) and Wavelet Transform (WT). K-nearest neighbor model with features obtained with FFT sustained its performance continuously with a 0.56 cross validation value.