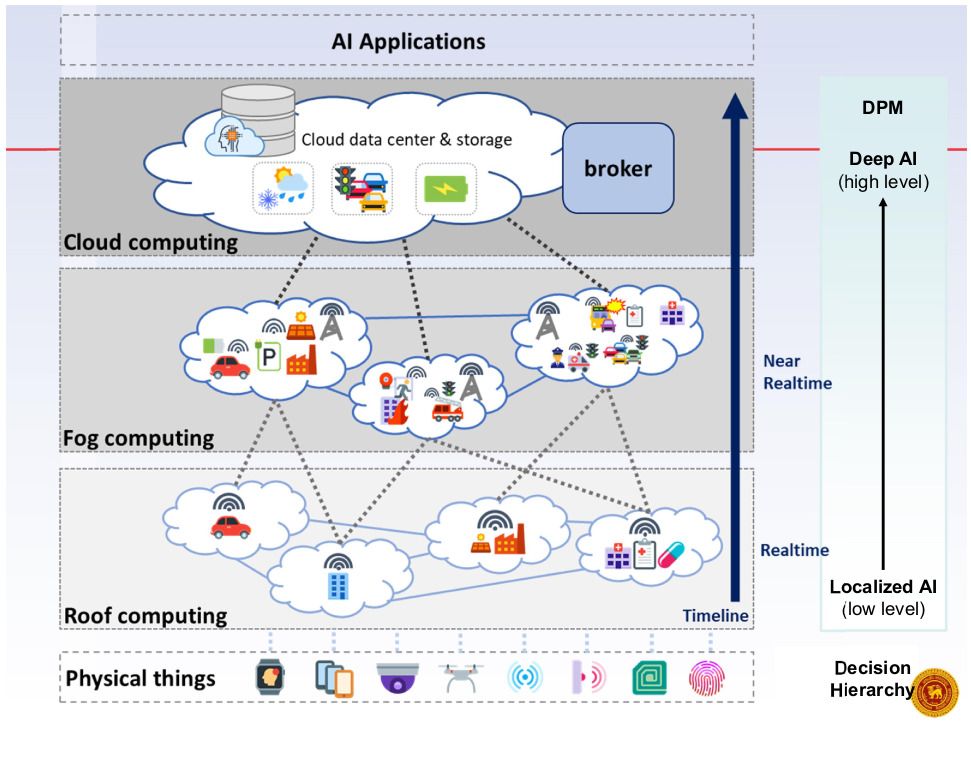
Supervisors
Authors
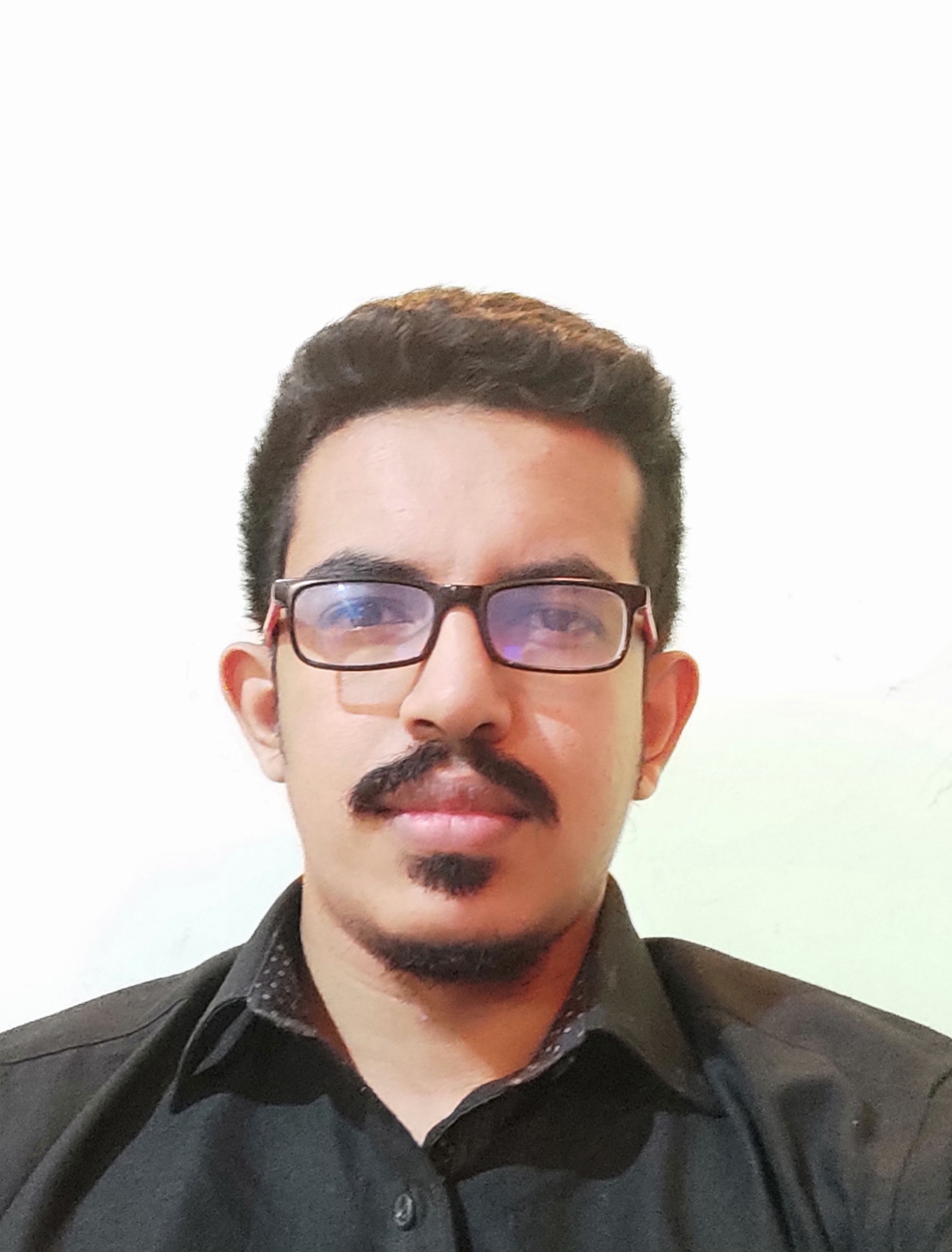
S.P.A.P.E Weerasinhge
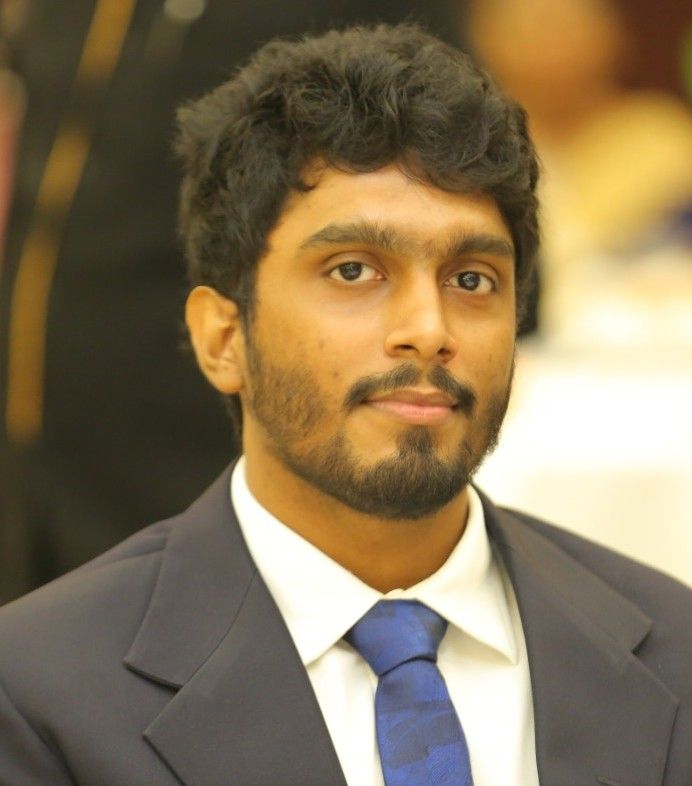
R.L Opanayaka
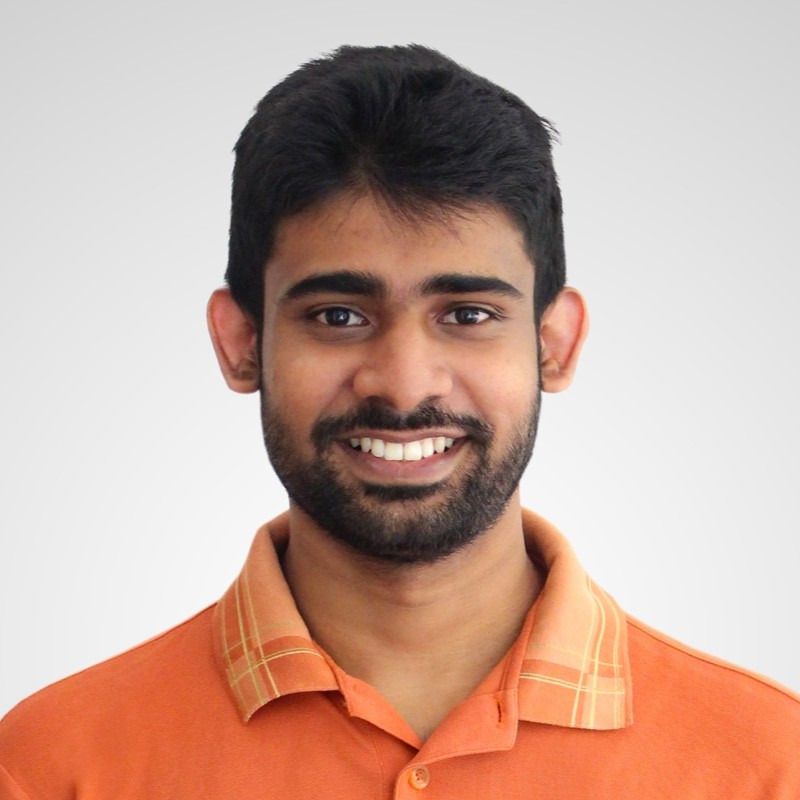
Artificial Intelligence has impacted in a variety of industries, leading the world towards revolutionary applications and services that are primarily driven by high-performance computation and storage facilities in the cloud. This is mainly due to the advantage of having higher computational power, larger storage capacity and scalability. But with the increase of millions of IoT devices, a huge amount of data is being generated by end devices. To process such data, the distributed end devices have to communicate with the cloud servers making it difficult to generate real-time decisions though it consumes a lot of resources including bandwidth, processing power, and storage facilities at the cloud. On the other hand, Edge computing architectures enable a distributed way to process data near the sources of data which leads to facilitate real-time processing. But with the limited resources in the end devices, it is quite challenging to perform complex AI algorithms. Hence to facilitate such services and to enable real time processing at the edge, a novel approach is proposed base on distributed computation, vectorization, computation offloading, parallelization, and federated learning techniques.